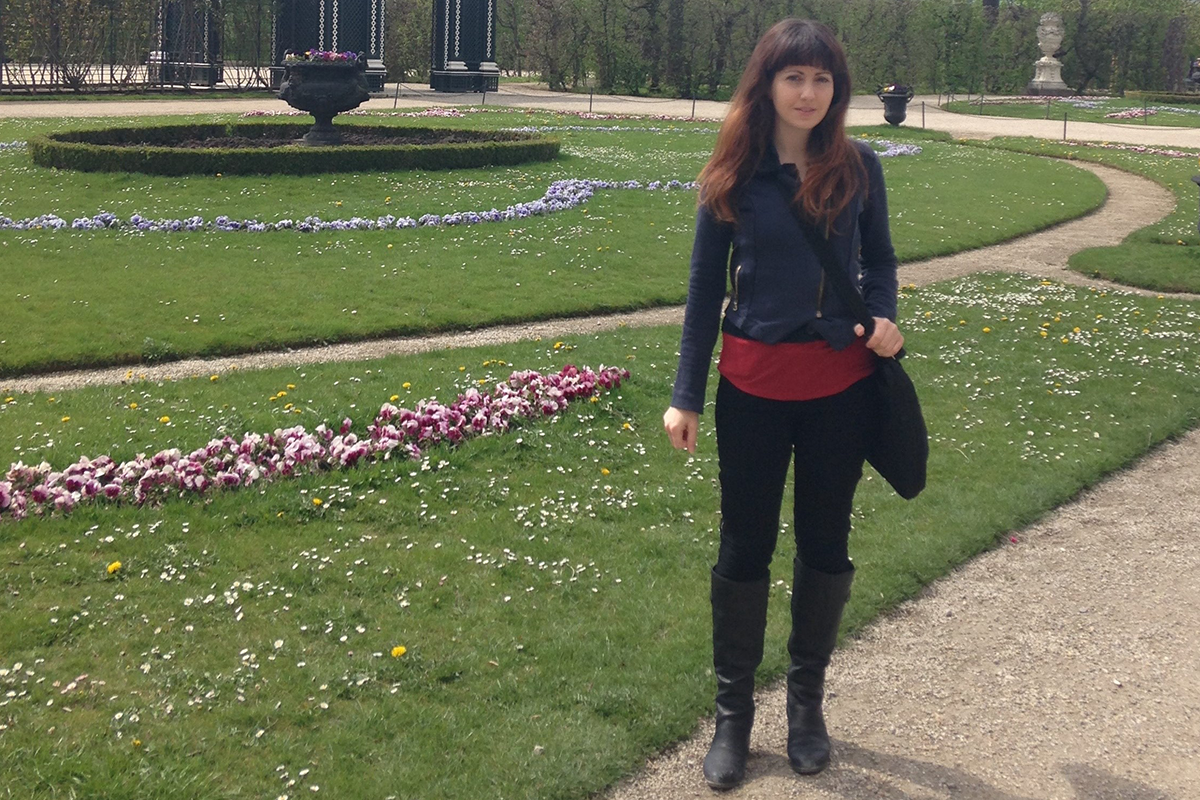
A model underway uses a novel mix of complex physics and machine learning to unravel how ice crystals grow in climate-consequential cirrus clouds
Kara Lamb is a researcher in the Department of Earth and Environmental Engineering at Columbia University. She is also principal investigator on a project at a center called Learning the Earth with Artificial Intelligence and Physics (LEAP), funded by the National Science Foundation (NSF).
After briefly considering a career studying literature, Lamb’s love of physics won out during her undergraduate years at the University of Illinois Urbana-Champaign (B.S., physics, 2007). On the way to atmospheric science, her interests led her to pursue physics graduate work at the University of Chicago (M.S. 2008, PhD 2015).
When she was first there, Lamb took a two-year deep dive into working on experimental atomic, molecular, and optical physics—an adventure in “quantum computation,” she says.
She soon steered into what she saw as a more practical pursuit—inquiries into the physics of the atmosphere.
“I was searching for a research direction,” says Lamb, when her mother, a geologist, suggested looking into geophysics.
From there, Lamb worked on her dissertation on ice cloud microphysics in the lab of geophysical sciences associate professor Elisabeth Moyer, whose group focuses on in situ atmospheric water vapor measurements.
“I wanted to do something that felt like a pressing challenge,” says Lamb. “And I couldn’t think of anything more pressing than understanding the climate.”
Influential Ice Crystals
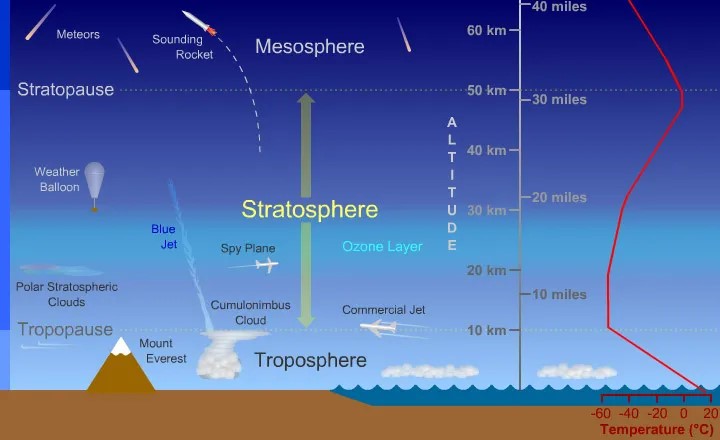
To that end, part of Lamb’s research these days is a 2022—2025 investigation of depositional ice growth in cirrus clouds funded by the Atmospheric System Research (ASR) program at the U.S. Department of Energy (DOE).
Ice grows within clouds when temperatures are cold enough that water vapor condenses into ice crystals. These crystals either form on their own or build up around bits of particulate matter, referred to as ice nucleating particles.
Ice deposition is the layer-by-layer growth caused by water molecules depositing onto an ice crystal surface. It is a critical process in the evolution of cirrus clouds, the wispy, pure-ice clouds that form at high altitudes. The kinetics of surface attachments that control water vapor being deposited on growing ice crystals are complex, hard to observe, and hard to predict.
Yet the growth rates and habits (shapes) of these crystals “influence the radiative effects of clouds—how they scatter and absorb light from the sun,” says Lamb. “Ice microphysics is a major source of uncertainty in climate models.”
“Microphysics” refers to small-scale physical processes that affect large-scale phenomena. In clouds, microphysical processes that determine how ice crystals grow and evolve in the atmosphere are little-known in detail. Depositional ice growth is one of these processes.
Into the Stratosphere
Joining Lamb in the ASR project are co-investigators Jerry Y. Harrington at Pennsylvania State University and Marcus van Lier-Walqui of Columbia University and the NASA Goddard Institute for Space Studies.
Harrington was a co-author of a 2023 paper, led by Lamb, that compared different proposed models for depositional ice growth with cloud-chamber observations of ice growing in conditions characteristic of the high-altitude cirrus clouds that form in the upper troposphere. (A paper describing part 2 of the study, which will also credit ASR, is still in preparation.)
Ultimately, Lamb and her co-investigators are trying to model the “fundamental microphysical processes” governing ice formation in high-altitude cirrus clouds, she says—to simulate how ice clouds evolve and change in the atmosphere.
Cirrus clouds that are important in climate terms form high up in the tropics, says Lamb, since they become “really important for regulating how much water enters the stratosphere.”
The stratosphere, where the concentrations of water vapor are in an absolute sense very low, is the layer of the atmosphere capped at about 30 miles above the surface of the Earth, more than halfway to the edge of space.
Investigating ice crystal growth is not only important because of ice’s cloud-radiative effects, but also to understand how efficiently ice crystals take up water. Cirrus clouds that form in the upper troposphere represent the last stage of dehydration for air that enters the stratosphere from the troposphere.
Once in the stratosphere, says Lamb, “water vapor acts like an important greenhouse gas.”
Observational Limits
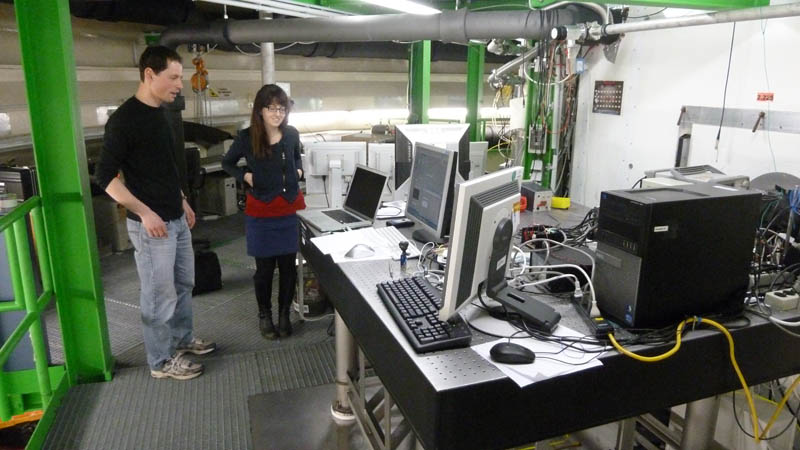
There is a lack of clear physical understanding of how ice crystals grow, in part because “there are limitations and challenges with all the different observational methods,” says Lamb.
It is possible to observe how a single ice crystal grows in a laboratory diffusion chamber and what habit it takes on. (Harrington’s group at Penn State does this kind of single-crystal observation work.)
But such data do not deliver “the full picture of how crystals behave in a cloud,” says Lamb.
During her PhD, Lamb participated in experiments at the Aerosol Interactions and Dynamics in the Atmosphere (AIDA) aerosol and cloud chamber at the Karlsruhe Institute of Technology in Germany, where it is possible to create realistic cirrus cloud conditions. But in terms of the growth rate of a single crystal, observations of ensembles of ice crystals growing in a cloud chamber are challenging to interpret.
And observations from aircraft don’t provide a timeline of ice crystal growth—the morphological evolution an ice crystal undergoes.
That’s where a model comes in—that is, a mathematical description of the ice growth process.
A model based on the mechanisms behind the growth rate of a single ice crystal, Lamb says, would improve our understanding of how ice clouds evolve and change in the atmosphere.
AIDA and IsoCloud
Lamb’s PhD dissertation work focused on studying isotopic physics in conditions characteristic of cirrus cloud formation in the atmosphere. This fundamental research was needed to interpret atmospheric observations of the isotopic composition of water vapor, which can be used as a tracer of the history of air in the atmosphere.
In that work, she developed a tunable diode laser absorption spectrometer instrument called the Chicago Water Isotope Spectrometer (Chi-WIS) to measure water and its isotopic composition.
With the Chi-WIS in hand, Lamb participated in a 2013 laboratory campaign at AIDA called IsoCloud and developed a model to constrain the fundamental processes governing how the isotopic composition of water vapor would evolve during ice cloud formation.
Just months after finishing a PhD, she signed on as a research scientist in the Chemical Sciences Division at the Cooperative Institute for Research in Environmental Sciences (CIRES) in Colorado. She wanted to get hands-on experience participating in aircraft field campaigns, and the position at CIRES gave her the opportunity.
Physics-Informed Machine Learning
Lamb and her ASR project teammates were motivated in part by the fact that the shapes of ice crystals and how fast they grow are not well predicted in weather and climate models. This is especially true in high-altitude cirrus clouds, where the surface processes that control ice deposition are not well understood.
The team took advantage of recent advances in the Diffusion Surface Kinetics Ice Crystal Evolution (DiSKICE) model developed by Harrington’s group, which allows for more complicated shapes to be modeled in single crystals. From there, Lamb and collaborators are combining measurements from a cloud chamber, field observations from the Harrington-led 2022 Project IceBALL field campaign (using a new balloon-borne ice crystal sampling system), and a large data set of crystals grown in the laboratory to derive constraints on ice growth models needed to simulate cirrus clouds.
Project IceBALL, formally called Ice Cryo-Encapsulation by Balloon, took place over three weeks at the Southern Great Plains atmospheric observatory in Oklahoma and was sponsored by DOE’s Atmospheric Radiation Measurement (ARM) user facility.
In the end, the ASR team hopes to emerge with better parameterizations of vapor deposition of ice crystals at very low temperatures, surface kinetics, ice nucleation, and crystal density. (Parameterizations are fine-scale models of microphysics that can be used in atmospheric models.) Lamb’s project through the LEAP center, in collaboration with researchers at NSF’s Colorado-based National Center for Atmospheric Research (NCAR), plans to bring these new parameterizations to the Community Earth System Model.
Lamb and the others are developing a model of ice crystal deposition in cirrus clouds by blending complicated physics with Bayesian probability statistics and a class of deep-learning models called neural ordinary differential equations (NODES).
NODES are deep-learning models that can be used to simulate dynamical systems like depositional ice growth, but without a priori assumptions about the functional dependence of unobserved physical processes.
Aircraft Ice Crystal Data sets
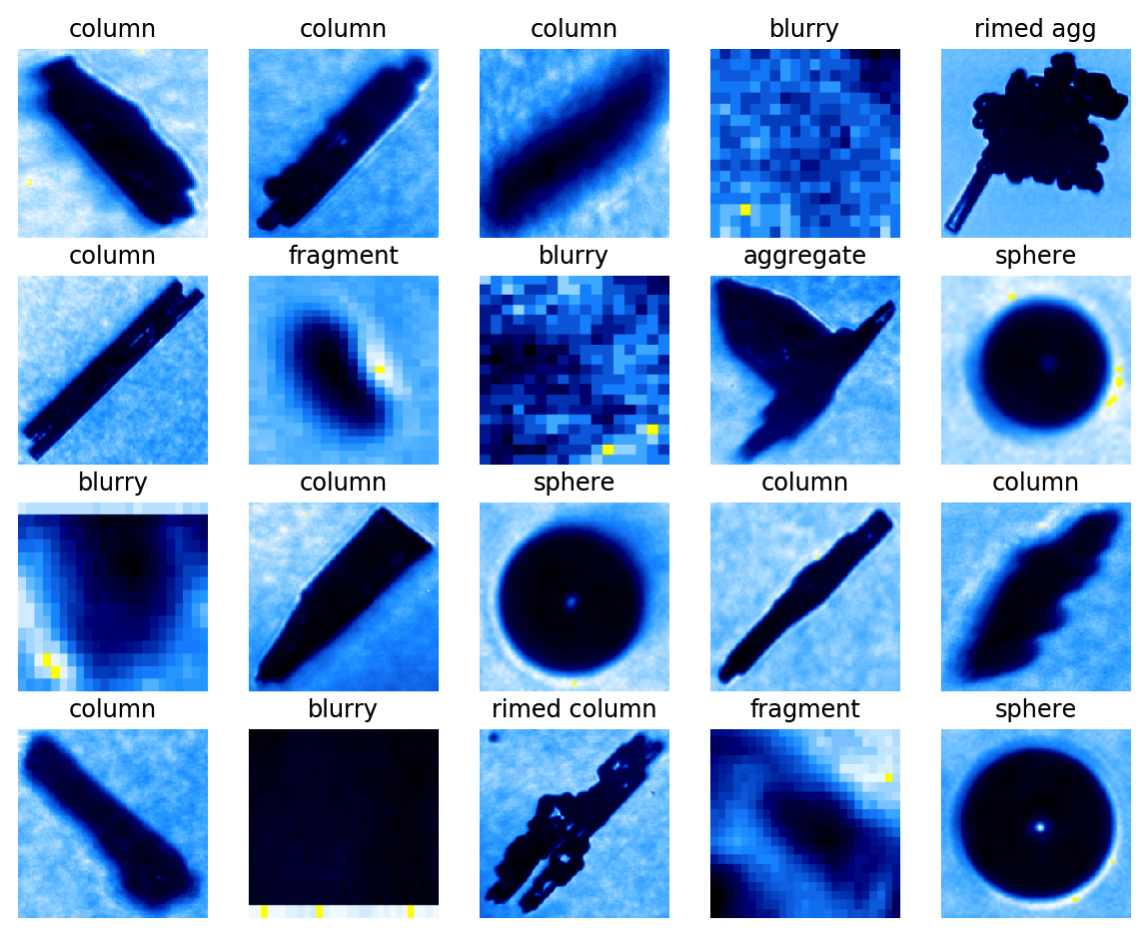
Lamb and her group are also employing unsupervised machine learning methods to study the diversity of ice crystals observed in aircraft data sets from cloud particle imager probes. (Unsupervised machine learning methods uncover patterns in data without the need for human intervention.)
This work draws on an ASR-funded database of ice crystal shapes called COCPIT, or Classification of Cloud Particle Imagery and Thermodynamics. It was assembled at the University at Albany – State University of New York by ice-crystal growth theorist Kara Sulia and her research group.
That way, says Lamb, it’s possible to learn “how ice crystal habits develop and how the different shapes relate to one another.”
In addition, back at Columbia’s LEAP center, Lamb mentee and postdoctoral researcher Joseph Ko is using computer vision methods to reconstruct COCPIT’s 2-dimensional images into 3-dimensional versions. The goal is to improve the understanding of ice crystal mass-dimension relationships, which will be used to improve ice microphysics representations in atmospheric models, such as NCAR’s Community Earth System Model.
Thinking of Observations in New Ways
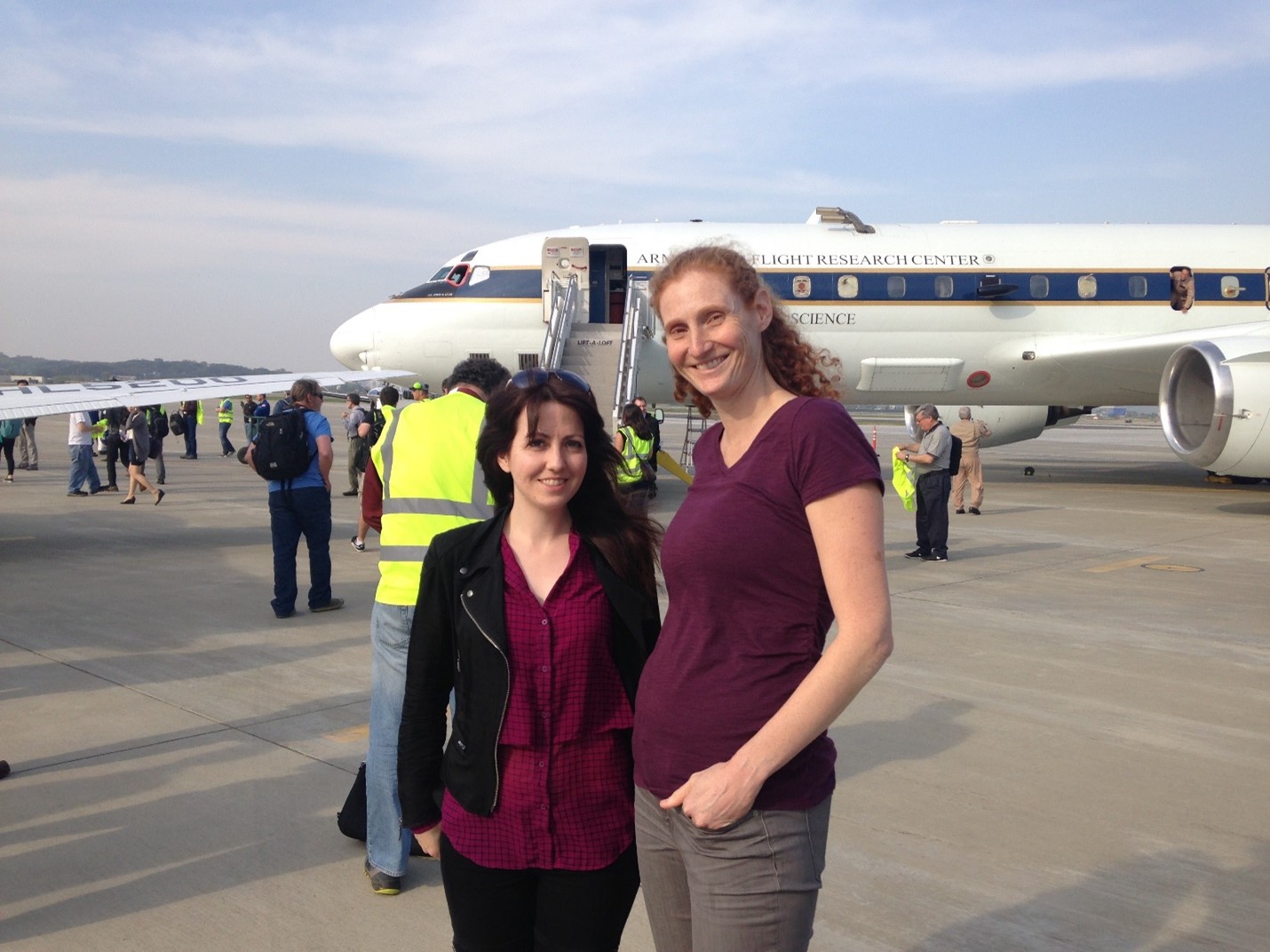
Lamb’s time at CIRES (2016─2020) was one of “being around so many people studying the composition of the atmosphere,” she says. It also led to her interest in the potential for applying machine learning methods to the atmospheric sciences.
Her research there focused on observing black carbon aerosols with the single-particle soot photometer (SP2), an instrument that enables black carbon to be measured in single aerosol particles.
In 2016, Lamb joined her CIRES mentor, Joshua Schwarz, and research scientist Anne Perring (now a professor at Colgate University), in the Korea-United States Air Quality (KORUS-AQ) field study in South Korea. KORUS-AQ deployed observational assets on land, ocean, and airborne platforms.
After that, Lamb took part in a joint NOAA-NASA venture called the Fire Influence on Regional to Global Environments and Air Quality (FIREX-AQ) Firelab Study at the Missoula (Montana) Fire Sciences Laboratory. The study focused on black and brown carbon aerosol optics in emissions from fuels characteristic of Western U.S. wildfires.
Later, she looked at SP2 observational data from the ATom data sets, which sparked her interest in machine learning.
“ATom” stands for the Atmospheric Tomography Mission, a 2016─2018 global-scale, four-season tropospheric sampling campaign flown by the NASA DC-8 research aircraft that made measurements of ozone, methane, and black carbon aerosols. Such aerosols influence both air quality and global warming.
In March 2020, Lamb moved on to Columbia, in part to learn more about the “state-of-the-art approaches people are using in scientific machine learning and how they are being applied to atmospheric research,” she says.
She has since worked on several studies using machine learning to improve cloud and convection parameterizations for climate models.
Before long, Lamb became curious about a data set on single-particle ice crystal images, which was a return to her PhD work simulating ice formation in cirrus clouds.
From there, she says, the totality of such projects means that much of her time is spent asking, “How can we think of observation in new ways, in order to inform our models?”
# # #This work was supported by the U.S. Department of Energy’s Office of Science, through the Biological and Environmental Research program as part of the Atmospheric System Research program.